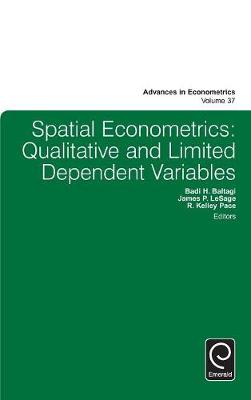
Stock image for illustration purposes only - book cover, edition or condition may vary.
Spatial Econometrics: Qualitative and Limited Dependent Variables
Badi H. Baltagi (Ed.)
€ 166.43
FREE Delivery in Ireland
Description for Spatial Econometrics: Qualitative and Limited Dependent Variables
Hardback. Advances in Econometrics 37 highlights key research in econometrics in a user friendly way for economists who are not econometricians. Editor(s): Fomby, Thomas B.; Escanciano, Juan Carlos; Hillebrand, Eric; Jeliazkov, Ivan; Pace, R. Kelley; Hill, R. Carter; Baltagi, Badi H.; LeSage, James P. Series: Advances in Econometrics. Num Pages: 408 pages. BIC Classification: KCH. Category: (G) General (US: Trade). Dimension: 162 x 240 x 27. Weight in Grams: 672.
Advances in Econometrics is a research annual whose editorial policy is to publish original research articles that contain enough details so that economists and econometricians who are not experts in the topics will find them accessible and useful in their research. Volume 37 exemplifies this focus by highlighting key research from new developments in econometrics.
Advances in Econometrics is a research annual whose editorial policy is to publish original research articles that contain enough details so that economists and econometricians who are not experts in the topics will find them accessible and useful in their research. Volume 37 exemplifies this focus by highlighting key research from new developments in econometrics.
Product Details
Publisher
Emerald Publishing Limited
Format
Hardback
Publication date
2016
Series
Advances in Econometrics
Condition
New
Number of Pages
408
Place of Publication
Bingley, United Kingdom
ISBN
9781785609862
SKU
V9781785609862
Shipping Time
Usually ships in 7 to 11 working days
Ref
99-50
About Badi H. Baltagi (Ed.)
Badi H. Baltagi, Syracuse University, Syracuse, NY, USA James P. Lesage, Texas State University, San Marcos, TX, USA R. Kelley Pace, Louisiana State University, Baton Rouge, LA, USA
Reviews for Spatial Econometrics: Qualitative and Limited Dependent Variables
Seven of the eleven papers in this collection explain how to estimate discrete dependent variables with spatial dependence using maximum likelihood and how to estimate binary and count dependent variables using Bayesian methods. A generic algorithm for numerically accurate likelihood evaluates spatial models characterized by a high-dimensional latent Gaussian process and non-Gaussian response variables. The remaining four papers address continuous ... Read more