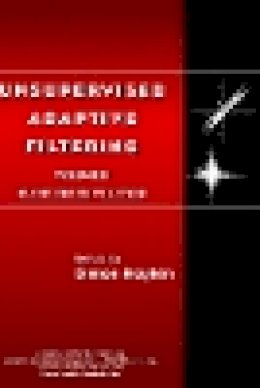
Stock image for illustration purposes only - book cover, edition or condition may vary.
Unsupervised Adaptive Filtering
Simon Haykin
€ 146.60
FREE Delivery in Ireland
Description for Unsupervised Adaptive Filtering
Hardcover. A complete, one-stop reference on the state of the art of unsupervised adaptive filtering While unsupervised adaptive filtering has its roots in the 1960s, more recent advances in signal processing, information theory, imaging, and remote sensing have made this a hot area for research in several diverse fields. Series: Adaptive and Learning Systems for Signal Processing, Communications and Control Series. Num Pages: 200 pages, Ill. BIC Classification: TJF; TJK. Category: (P) Professional & Vocational; (UP) Postgraduate, Research & Scholarly; (UU) Undergraduate. Dimension: 243 x 167 x 18. Weight in Grams: 454.
A complete, one-stop reference on the state of the art of unsupervised adaptive filtering
While unsupervised adaptive filtering has its roots in the 1960s, more recent advances in signal processing, information theory, imaging, and remote sensing have made this a hot area for research in several diverse fields. This book brings together cutting-edge information previously available only in disparate papers and articles, presenting a thorough and integrated treatment of the two major classes of algorithms used in the field, namely, blind signal separation and blind channel equalization algorithms.
Divided into two volumes for ease ... Read more
A complete, one-stop reference on the state of the art of unsupervised adaptive filtering
While unsupervised adaptive filtering has its roots in the 1960s, more recent advances in signal processing, information theory, imaging, and remote sensing have made this a hot area for research in several diverse fields. This book brings together cutting-edge information previously available only in disparate papers and articles, presenting a thorough and integrated treatment of the two major classes of algorithms used in the field, namely, blind signal separation and blind channel equalization algorithms.
Divided into two volumes for ease ... Read more
Product Details
Format
Hardback
Publication date
2000
Publisher
John Wiley and Sons Ltd United States
Number of pages
200
Condition
New
Series
Adaptive and Learning Systems for Signal Processing, Communications and Control Series
Number of Pages
200
Place of Publication
, United States
ISBN
9780471379416
SKU
V9780471379416
Shipping Time
Usually ships in 7 to 11 working days
Ref
99-1
About Simon Haykin
SIMON HAYKIN, PhD, is University Professor and Director of the Adaptive Systems Laboratory at McMaster University.
Reviews for Unsupervised Adaptive Filtering